September 28, 2020
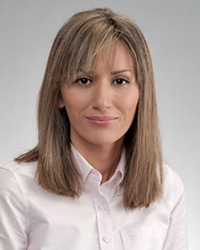
Roxana Darvari
Sizing Stormwater Control Measures for Edwards Aquifer Pollution Mitigation
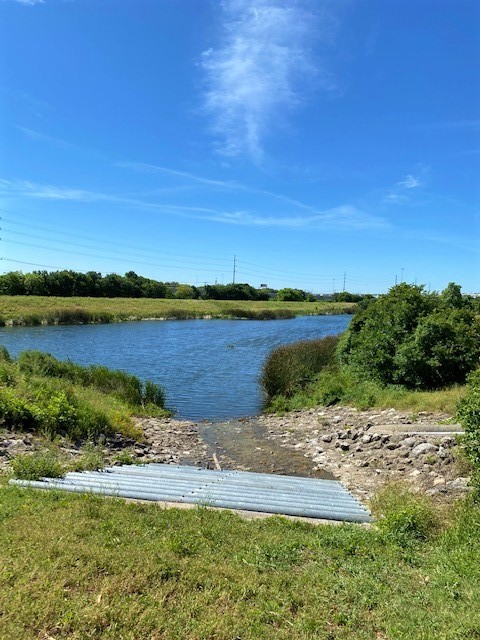
Stormwater management is integrated with land development to control surface water and groundwater quality, mitigate flood risk, and enhance community benefits. As rainfall runoff makes its way across urban landscapes, it carries oil, chemicals, construction waste, and other pollutants into surface and subsurface water bodies.
The karstic Edwards Aquifer is a critical Central Texas water resource. Land development around the aquifer is regulated by the Texas Commission on Environmental Quality through the Edwards Aquifer Protection Program. This regulation requires removal of 80% of the incremental increase in total suspended solids (TSS) from runoff that could quickly enter and pollute the aquifer.
This standard can be accomplished by implementing Best Management Practices (BMPs). BMPs are structural, vegetative, or managerial practices used to treat, prevent, or reduce water pollution. We have developed a TSS load calculator based on a probabilistic method, which uses long-term hourly rainfall records, for sizing the structural BMPs to verify that proposed construction will meet Edwards Aquifer regulations. We have also provided a technical guidance document for use by engineers, land planners, and developers to help them meet TSS reduction requirements for stormwater runoff.
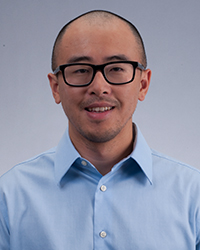
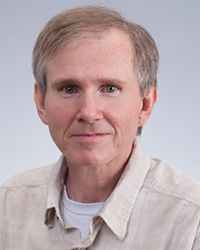
Jinyu Zhang & William Ambrose
Training Convolutional Neural Networks to Identify Lithofacies of ~2,000 ft Cores in the Permian Basin
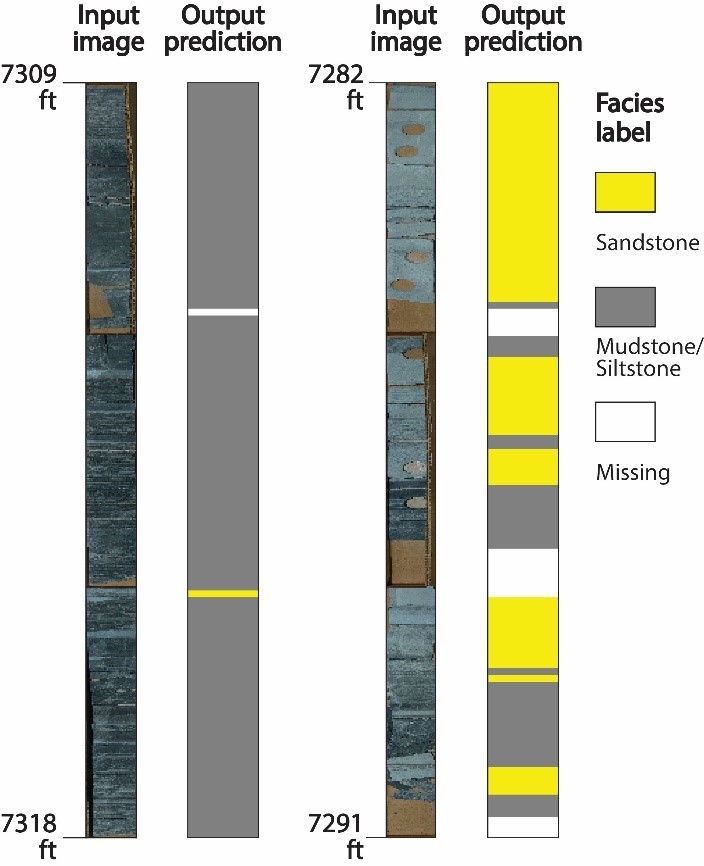
Input core images and core facies predicted by the deep-learning model.
Convolutional neural networks (CNNs), one of the most widely employed deep learning techniques, have achieved great success in image recognition. However, CNNs are less widespread in sedimentary studies, partially because it is challenging to generate a large-scale training database for sedimentary data.
We are developing and testing a CNN model for defining lithofacies in the Permian Basin. The Permian Basin is an ideal laboratory because of its huge hydrocarbon potential and rich dataset. We have compiled ~20,000 image samples with labeled facies from ~2,000 ft of whole cores in the Permian Basin. This database is used to train and evaluate a CNN model predicting lithofacies from core images.
The best model returns an accuracy of 0.83 when evaluated by an independent testing dataset. Accuracy increases when the number of samples increases. Even the learned model trained by 1% data (n = 200) can return 0.70 accuracy. These results suggest the deep-learning model can rapidly provide analysis of core data and can be used to accelerate core descriptions. A large-scale database covering different depositional environments is critical in applying artificial intelligence in lithofacies detection.
With access to 2 million boxes of core and deep expertise in sedimentary analysis, research groups at Bureau of Economic Geology have an advantage in building a core training database for deep-learning models.