Shuvajit Bhattacharya
About Shuvajit Bhattacharya
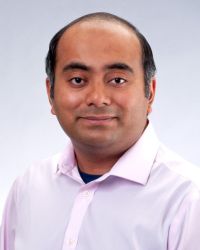
Dr. Shuvajit Bhattacharya is a petrophysicist and geophysicist at the Bureau of Economic Geology. Currently, Shuvajit is involved in multiple research projects on unconventional resources, geothermal energy, carbon, and hydrogen storage in the subsurface. He has a Ph.D. in geology focused on machine learning and petrophysics from West Virginia University (2016) and an M.Sc. in applied geophysics from the Indian Institute of Technology Mumbai (2010). Shuvajit is the recipient of the Society of Exploration Geophysicists J. Clarence Karcher Award (2022) for “significant contribution to science and technology of exploration geophysics by a young geophysicist of outstanding abilities.”
What are your current research activities?
I am primarily interested in advancing the science and technology of petrophysics and seismic to improve our current understanding of subsurface rock types, rock, and fluid properties at regional and local scales. My current research activities involve developing core-calibrated petrophysical models, quantitative seismic interpretation, and machine learning for integrated subsurface characterization and time-lapse monitoring.
I have completed multiple research projects related to hydrocarbon resources exploration from conventional and unconventional reservoirs, carbon sequestration, geothermal energy, and hydrogen storage, which are needed for reliable energy supply and mitigating climate change. As a result, I have contributed to different research groups at the Bureau of Economic Geology.
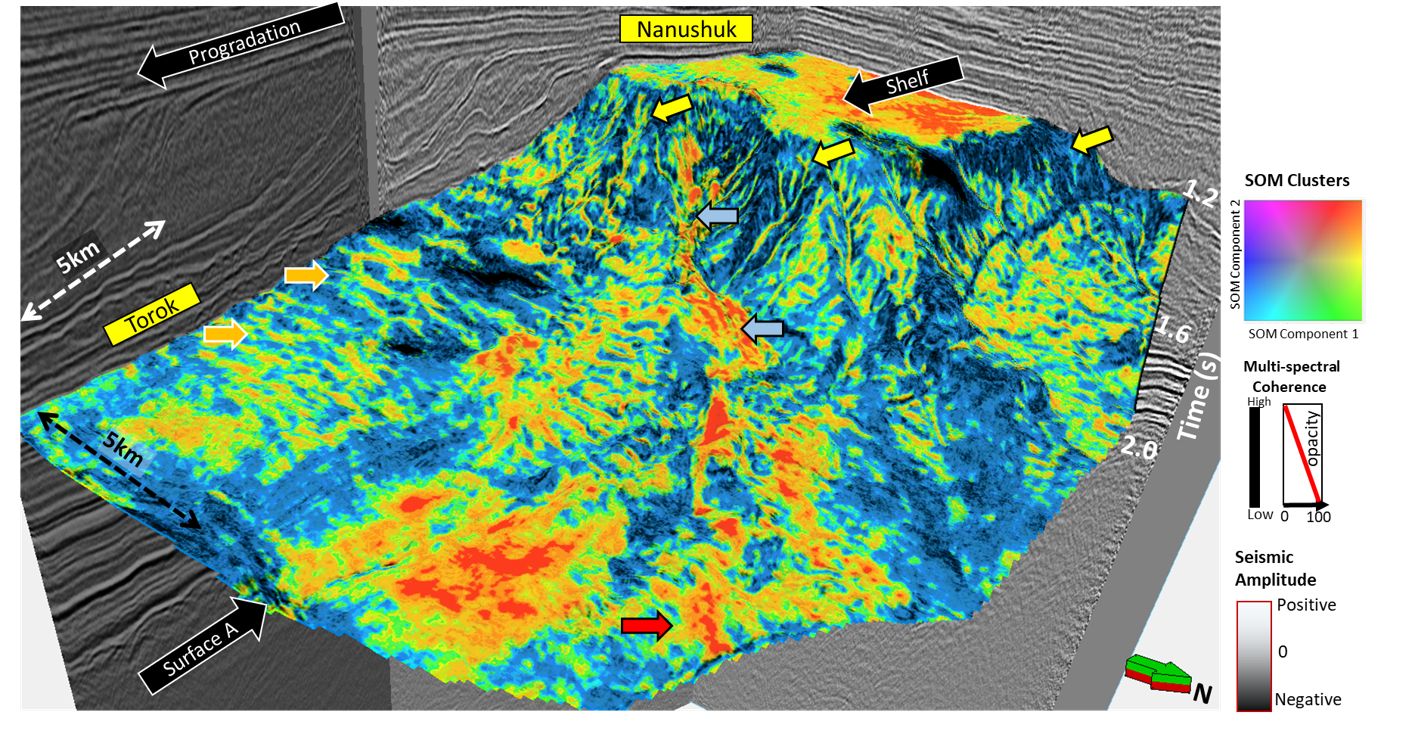
Unsupervised machine learning and seismic attribute-based 3D seismic interpretation (Bhattacharya and others, 2022). Arrows indicate shelf, canyon, basin-floor fan, and sediment wave deposits. Data courtesy: 2017: State of Alaska, Department of Natural Resources, Division of Oil & Gas, AS 43.55 Exploration Tax Credit Project. http://dog.dnr.alaska.gov/Information/GeologicalAndGeophysicalData
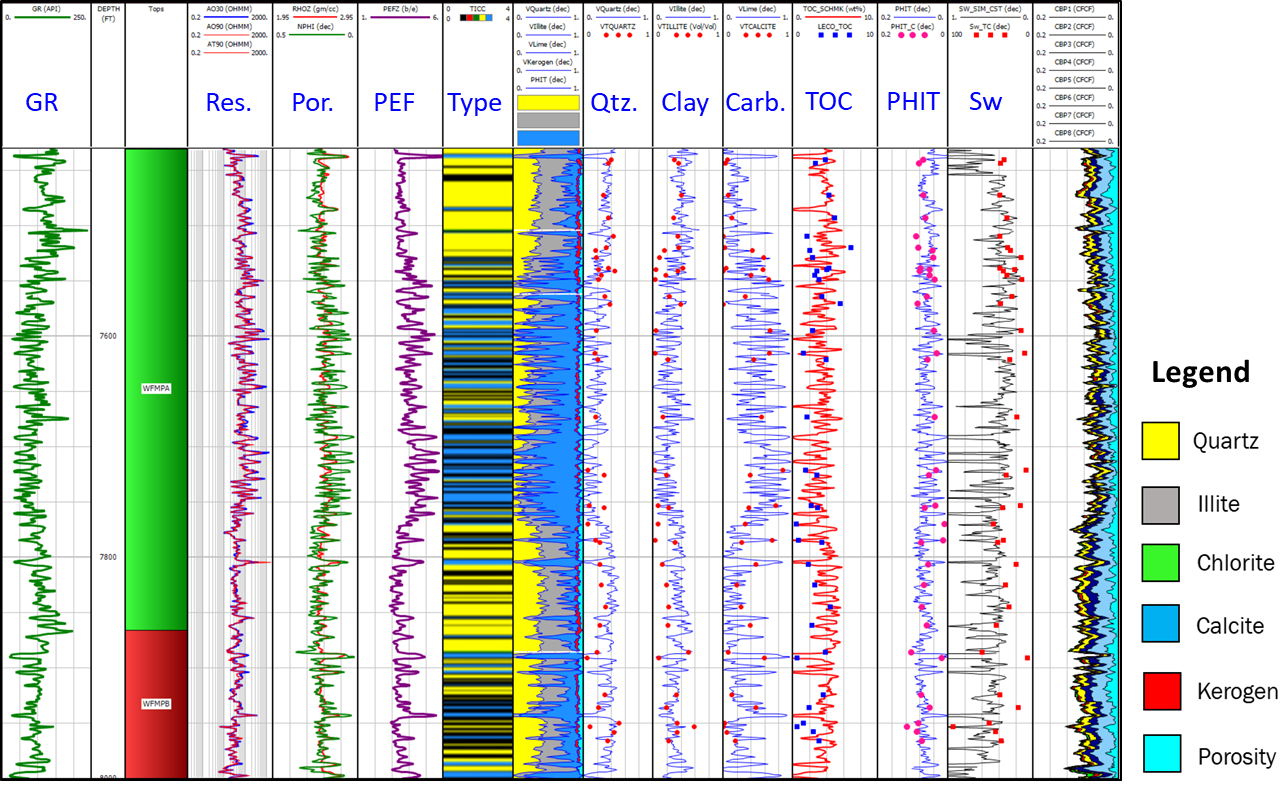
An example of a core-calibrated petrophysical evaluation of a mudrock reservoir (Bhattacharya and others, 2022).
What excites you the most about your current research?
I enjoy observing the petrophysical and seismic “wiggles” on screen or paper and analyzing their patterns to decipher what they can tell us about a billion years of Earth’s history and resources and validating those with ground truth information. I like working on a range of collaborative, integrated projects. As a researcher at the Bureau, it is equally challenging, joyful, and rewarding to work on subsurface characterization using well logs from hundreds to thousands of wells and integrate those analyses with core, often complemented by the Austin Core Research Center, located next to the Bureau.
I am also excited by the new developments in machine learning for efficient subsurface data analytics as well as the role of petrophysics and seismic (especially from subsurface monitoring aspects) in the upcoming energy mix, such as carbon and hydrogen storage and geothermal energy. It is a great opportunity to push the boundaries of seismic and petrophysical applications beyond hydrocarbon exploration.
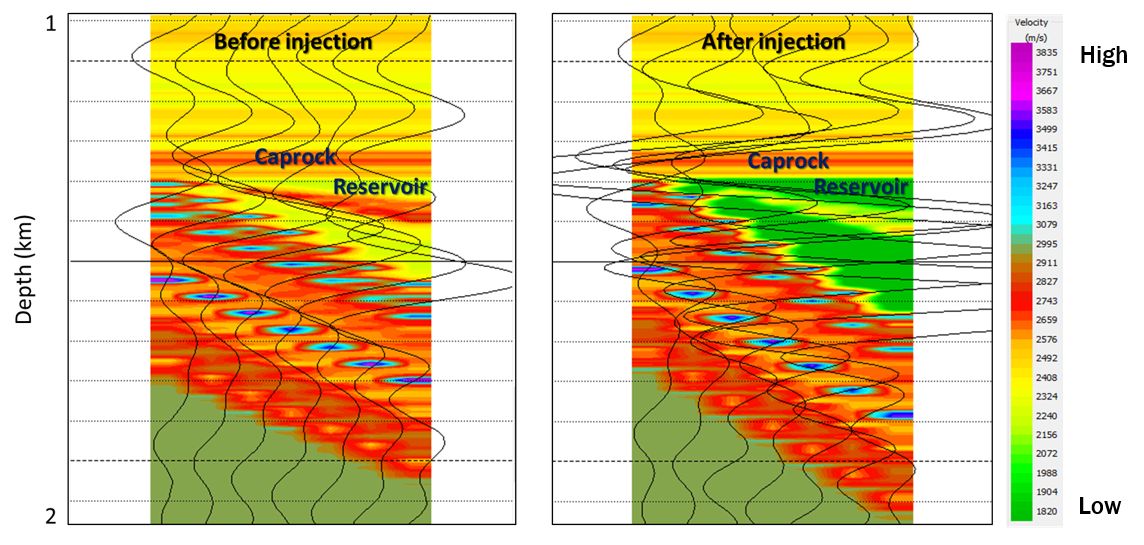
Changes in seismic response due to hydrogen storage in the subsurface (Bhattacharya, 2022).
What is the desired outcome of your research?
Improve our understanding of the subsurface in a quantitative manner and identify key controls on rock properties relevant to fluid storage and recovery, including hydrocarbons, CO2, and hydrogen. I would also like to see diversified applications of borehole geophysics and petrophysics beyond oil and gas, such as in carbon and hydrogen storage, critical minerals research, and non-energy-related academic research.
What do you need in order to make your research efforts more successful?
I am actively looking for advanced petrophysical logs (open hole and cased hole) and core data from energy companies or other kind of data providers. 3D pre-stack seismic data will be of great help! These data will help me develop new methods for subsurface characterization and test and validate research ideas for a range of projects.
Strong partnerships with national and international funding sponsors and professional geoscientists working in the industry, as well as national labs, will make my research more impactful.
What are your latest papers or publications, and what is most exciting about them?
Bhattacharya, S., 2022, Unsupervised time series clustering, class-based ensemble machine learning, and petrophysical modeling for predicting shear sonic wave slowness in heterogeneous rocks: Geophysics, v. 87, no. 5, p. D161–D174, https://doi.org/10.1190/geo2021-0478.1.
Shear wave velocity is critical for formation evaluation, rock physics, and quantitative reservoir characterization. In this study, I discussed the fundamental drawbacks of traditional machine learning approaches in geosciences and proposed new approaches to machine learning, namely, unsupervised multivariate time series clustering (Toeplitz inverse covariance-based clustering) and class-based ensemble machine learning. I developed and integrated these tools with geologic information, petrophysical models, mineralogy, and in situ stress to predict shear wave velocity and Poisson’s ratio of the Wolfcamp Formation. Unlike the traditional approaches, my proposed approach honors geologic pattern and considers several fundamental natures of geologic data sets, such as attribute interdependence, temporal or depth dependence of attribute responses, and multimodal geologic factors, etc. The results from the proposed approach outperformed traditional machine learning approaches and commercially available AutoML (automated machine learning) tools in a large study area, by several orders of magnitude. Ultimately, the results were used to delineate brittle and ductile units in the mudrock formation for hydraulic stimulation.
Bhattacharya, S., Bakhshian, S., Hovorka, S., Uroza, C., Hosseini, S., Bump, A., Treviño, R., Olariu, I., and Haagsma, A., 2023, Integrated petrophysical studies for subsurface carbon sequestration: Society of Petrophysicists and Well Log Analysts’ Annual Logging Symposium Transactions.
Currently, many carbon capture and storage (CCS)-related petrophysical studies are limited to porosity and permeability for storage capacity and injectivity; however, there are several scientific questions and environmental regulations that need a thorough petrophysical evaluation, which we focused on in this study. Based on our review of many past projects, their impacts, as well as current approaches and needs, we proposed that the science-based basin-wide exploration, prospect identification, and maturation approach that is so prevalent in the oil and gas sector should be applied to carbon storage as well, as opposed to a few injection-site-specific characterization efforts. The study offers lessons learned from multiple case studies, such as the Miocene and Wilcox formations on the Gulf Coast, Texas, and the fractured Trenton–Black River groups in Michigan, showing pertinent problems where petrophysics can help facilitate successful CCS operations. Using simplified reservoir simulations, conventional and advanced petrophysical logs, and workflows, the study shows the impact of formation water salinity, pore pressure, and fractures on CO2 storage capacity and plume migration. The study also demonstrates how existing and emerging petrophysical workflows and downhole logging technologies can be implemented in CCS as well as the need to develop new concepts and tools.
Bhattacharya, S., 2021, A primer on machine learning in subsurface geosciences: Cham, Switzerland, Springer Nature, SpringerBriefs in Petroleum Geosciences & Engineering, 182 p., ISBN 978-3-030-71767-4, https://doi.org/10.1007/978-3-030-71768-1.
In response to my publications, Springer requested that I write a book. This book is one of the first comprehensive books on machine learning for subsurface geoscientists. It provides a review and discussion of the fundamentals, workflow, proven success, promises, and perils of machine learning. It also provides a future trend of machine learning in the geosciences. It took me close to a year to complete this project, including several reviews, editing, and copyright permissions.
Who will benefit from your latest paper or publication?
Geoscientists working on quantitative seismic interpretation, rock physics, and reservoir characterization. The methods developed and implemented by me and the team would be applicable to several petrophysical, geophysical, and geomechanical studies in hydrocarbon, geothermal energy, carbon storage, and hydrogen storage studies.
What was your most exciting paper or publication and why?
Bhattacharya, S., Carr, T., and Pal, M., 2016, Comparison of supervised and unsupervised approaches for mudstone lithofacies classification: case studies from the Bakken and Mahantango–Marcellus shale, USA: Journal of Natural Gas Science and Engineering, v. 33, p. 1119–1133, https://doi.org/10.1016/j.jngse.2016.04.055.
Back in 2016, I published an article on the comparative performance of supervised and semi-supervised machine learning in petrophysical facies classification of complex mudrocks, specifically the Bakken and Marcellus shale, which was relatively new at that time. The multi-scale heterogeneous nature and large data dimensionality of mudrock reservoirs increase the challenge of quantitative facies interpretation by conventional core and outcrop-based descriptive methods. In this study, we developed and implemented a quantitative mudrock lithofacies classification approach (based on mineralogy and Total Organic Carbon) integrating core, geochemical data, and petrophysical logs, which are geologically meaningful, mappable, and predictable at core, well log, and regional scales. We showed that both Bakken and Marcellus shale members are vertically and laterally heterogeneous but can be classified into at least five mudstone lithofacies, along with calcareous siltstone and limestone lithofacies. We addressed several critical issues using quantitative lithofacies classification and machine learning-based prediction techniques from petrophysical logs, honoring geologic data and reduction of uncertainties.
This was one of the first quantitative mudstone lithofacies modeling studies that showed the heterogeneous nature of Bakken shale that can be modeled quantitatively. This is the first published study to show the comparative performance of different machine learning approaches to recognize complex mudrock lithofacies using petrophysical logs, which was implemented by several researchers worldwide.
Who are the types of research partners you are seeking, and what skills or expertise could be of benefit to your research?
I look forward to collaborating with geoscientists and reservoir engineers working in the energy industry, national labs, and academia. Specific research expertise in stratigraphy, geomechanics, geochemistry, seismic processing, and reservoir simulation would complement my research.
What are the desired relationships, expertise, or skills that could be brought in to benefit your research?
I seek partnerships with energy companies (domestic and international), private foundations, and national labs for data sharing, knowledge transfer, and giving us critical feedback on the overall direction and impact of applied research.
What have been recent successes associated with your research?
- Building basin-wide petrophysical models and pore pressure from hundreds of wells for the Wolfcamp and Spraberry formations in the entire Midland Basin for the Bureau’s Tight Oil Resource Assessment (TORA) program
- Geology and reservoir simulation-guided time-lapse surface and borehole geophysical modeling for CO2 and H2 plume monitoring for the Gulf Coast Carbon Center (GCCC), the Society of Exploration Geophysicists SEAM Foundation, and GeoH2
- Generation of benchmark 3D seismic data sets with full interpretations for training and testing various machine learning models for the Society of Exploration Geophysicists SEAM Foundation
- Building calibrated petrophysical models and maps at the Chicxulub crater at a mega-regional scale to estimate volumes of each lithology (e.g., anhydrite) that was vaporized or ejected due to the impact, in collaboration with the UT Institute for Geophysics’ researchers. This was a purely academic research project.
- Contributing to a multi-author, multi-institutional project report on the geology of the Texas-wide geothermal potential for the Mitchell Foundation
- Editing a book entitled Advances in Subsurface Data Analytics: Traditional and Physics-Based Machine Learning, published by Elsevier in 2022. This book brought together close to 40 experts from industry and academia from various parts of the world to produce a truly unique contribution to subsurface geosciences.
What is the geographic location of your research?
I primarily work on the Permian Basin and Gulf Coast of Texas. I have also worked on the North Slope (Alaska), Williston, Appalachian, and Michigan basins, and other countries, for example, Australia and the Netherlands, for a few research projects.