Upscaling high-resolution core-based facies to subsurface basin-scale stratigraphic models for the Wolfcamp and Third Bone Spring Sand, Delaware Basin. Application of machine learning workflows to geological studies.
Presenter
Toti E. Larson, Ph.D.
Research Scientist
Bureau of Economic Geology
Jackson School of Geosciences
The University of Texas at Austin
Description
Characterization of subsurface geological reservoirs is hindered by the high degree of heterogeneity and spatial variability of rock facies in sedimentary systems. This effort is especially difficult when trying to capture thin-bedded heterogeneity common to mudrock systems. Although core-based facies and rock attribute data sets provide the most direct measurements to inform static reservoir models, our ability to upscale high-resolution discrete core-based facies to the reservoir scale remains a challenge. A machine learning workflow to integrate high dimensionality, spatially complex, and multimodal core-based datasets with wireline logs is described for the Third Bone Spring Sand and Wolfcamp A and B units in the Delaware Basin of West Texas, United States. Model input parameters include categorical lithofacies core descriptions, X-ray fluorescence core scanning, and open-hole wireline logs. Core-based rock attribute data (e.g., X-ray diffraction mineralogy, total porosity, and total organic matter content) describe high-resolution core-based facies in the training datasets. Large volumes of open-hole triple combo wireline logs (gamma ray, deep resistivity, bulk density, and neutron porosity) are trained using these core-based training datasets to predict rock facies across the Delaware Basin to apply core-based facies classifications ‘beyond the core’ to the basin scale.
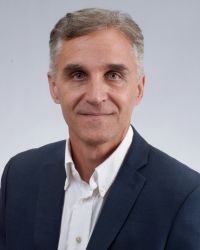