Nowcasting Soil Moisture with Satellite Data, In-Situ Measurements, and Deep Learning
H. Dashtian1, M.H. Young1, B.E. Young1, S.T. McKinney1, A.M. Rateb1, D. Niyogi2, S.V. Kumar3
1- Bureau of Economic Geology, Jackson School of Geosciences, The University of Texas at Austin
2- Dept. Earth and Planetary Sciences, Jackson School of Geosciences, The University of Texas at Austin
3- NASA Goddard Space Flight Center, Beltsville, MD
Study Summary
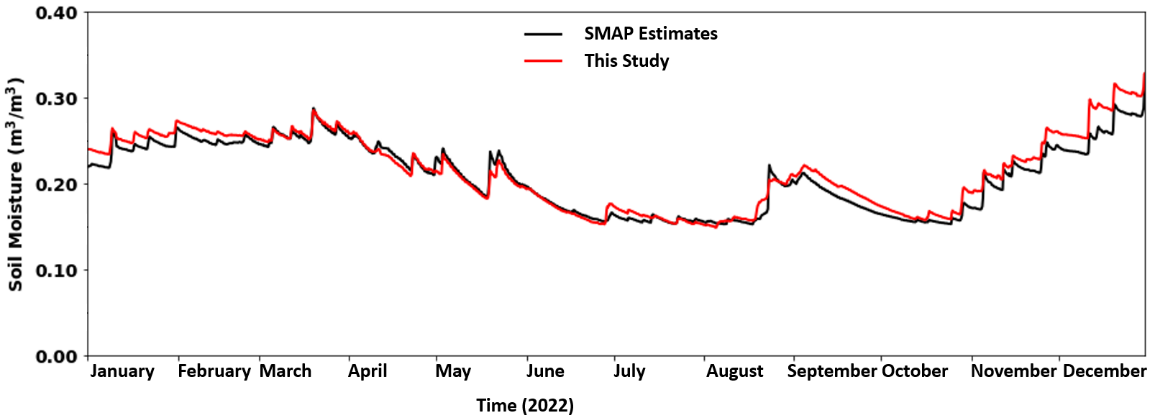
Soil moisture is a key component of the hydrologic cycle, moderating weather and climate patterns and flooding potential, as well as impacting agricultural production. Satellites from NASA and other agencies provide estimates of soil moisture at local and global scales, but data release is delayed by 3–5 days. This reduces our ability to estimate the current soil moisture conditions and thus future conditions, or to make decisions regarding risks from flooding or other events that are occurring right now. In this study, we sought to nowcast (to bring these delayed datasets to current time) NASA’s Soil Moisture Active Passive (SMAP) product. To accomplish this, we developed a model that integrates advanced machine learning techniques and different data sources, including field-based soil moisture observations collected from remote monitoring stations. We tested our approach over a large area (56,000+ km2) that includes Houston, Texas. Our methodology nowcasts soil moisture accurately in both time and space, addressing SMAP’s latency and offering near, real-time soil moisture estimates at high spatial resolution. The nowcasted data aligns closely with actual data released by NASA, capturing its variations in time and space.SMAP also underestimates the spatio-temporal variability of soil moisture compared to field-based data, highlighting the need to integrate different types of data. The proposed framework can improve real-time flood and drought monitoring, provide insights for various hydrological applications, and identify regions with higher uncertainties, guiding future model and data collection improvements.
Why is this research important and why do the results matter?
- Accurately estimating near-real-time soil moisture levels is a complex yet crucial task, especially in areas prone to natural soil moisture-related hazards, like floods, in the southeastern region of Texas.
- For the first time, we developed an approach that blends field-based and satellite data into accurate, current estimates of soil moisture, improving on the data typically released by NASA and other agencies.
- The method can be improved by using typical weather data as input, allowing us to nowcast soil moisture at larger scales, perhaps across the state of Texas.
- Real-time and forecasted soil moisture data are valuable in various applications, including irrigation management, and flood and fire risk assessment.
Full Reference: Dashtian, H., Young, M. H., Young, B. E., McKinney, S.T., Rateb, A. M., Niyogi, D., and Kumar, S. V., 2024. A Framework to Nowcast Soil Moisture with NASA SMAP Data Using In-Situ Measurements and Deep Learning: Journal of Hydrology: Regional Studies, v. 56, article 102020, doi:10.1016/j.ejrh.2024.102020.
Contact:
Hassan Dashtian, Ph.D., Bureau of Economic Geology, The University of Texas at Austin, hassan.dashtian@beg.utexas.edu