Improving prediction of subsurface reservoir properties using the power of geoscience, big data, and AI/ML methods
Presenter
Dr. Kelly Rose
Research Geologist
U.S. Department of Energy, National Energy Technology Laboratory
Abstract
Advancements in data science and artificial intelligence techniques have strong potential for improving analysis and prediction of porous media properties in subsurface reservoirs. Understanding geologic controls on porous media properties has implications for reducing the risks inherent in subsurface resource exploration, production, as well as underground storage of fluids such as CO₂ sequestration. The Subsurface Trend Analysis (STA) is a hybrid spatio-temporal geostatistical method for characterizing subsurface properties which provides a convenient artificial intelligence framework for complex, multivariate subsurface environments. The STA incorporates qualitative geologic knowledge and quantitative analyses of data in order to refine property and resource prediction. The STA can handle a range of data and scales, from sub-reservoir to basin-wide, to improve the prediction of subsurface properties at any scale. Applications of the STA have demonstrated positive basin- and regional-scale predictive capabilities when applied to reservoir properties. Integration of machine learning (ML) and natural language processing (NLP) techniques with the STA method are improving the prediction of subsurface properties via the use of broader-scale information to inform local, wellbore and reservoir-scale features.
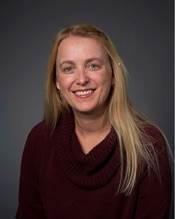