Impact of sedimentary facies on machine learning of acoustic impedance from seismic data: challenges and solutions
Presenter
Dr. Hongliu Zeng
Senior Research Scientist
Bureau of Economic Geology
The University of Texas at Austin
Description
This talk is focused on how to configure facies into machine learning (ML)-based seismic inversion. The main challenge is that most depositional systems have built-in complexities, which are difficult to describe in an ML model. Using a geologically realistic 3D model, I demonstrate that training score and prediction error can be correlated to facies pattern. ML with sparse wells is low score and highly unstable, which can be avoided by using a large synthetic training data set. Field-data tests show great potential to use ML in qualitative sand volume mapping, which can be helpful in studies of sedimentology, reservoir prediction, and CO₂ sequestration.
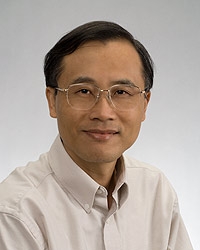