Deep Learning and Multivariate Time Series Clustering: Applications to Seismic and Petrophysical Interpretations
Presenter
Dr. Shuvajit Bhattacharya
Researcher
Bureau of Economic Geology, The University of Texas at Austin
Abstract
Machine learning (ML) is an emerging tool in geosciences. It holds some promises for efficient seismic and petrophysical data processing, integration, and interpretation. I will show two case studies on applying deep learning and time series clustering approaches to complex structural interpretation and rock property estimates.
The first case study is on interpreting a multi-phase normal fault network in the deep subsurface of the North Slope, Alaska. Such fault systems have controls on reservoir compartmentalization and resource recovery. 3D seismic attributes (e.g., curvature and aberrancy) and convolutional neural networks are used to classify the faults in an area of ~1,000 square kilometers. The results show the fault distributions along three different orientations, each connected to a tectonic event. The workflow and results presented can also be beneficial to the Permian Basin studies.
In the second case study, unsupervised time series clustering with adaptive windows is used to predict shear wave velocity in the Wolfcamp Formation in the Midland Basin. The availability of shear sonic logs is crucial to rock physics, geomechanics, and seismic modeling studies. However, such logs are often not publicly available. Time series clustering and class- based ensemble ML workflows can be used to predict shear sonic logs, Poisson’s ratio, andrigidity modulus for several wells in the basin. The results show that such rock properties can be estimated with high accuracy, at least at a local scale. The class-based ML approach helps constrain the model and makes it more interpretive.
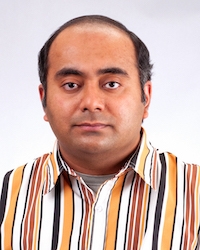