CorePy: Visualization and Neural Network Facies Models for Mudrock Systems
Core-based characterizations and analytical measurements provide critical information used to inform subsurface geologic models and ground-truth wireline-log measurements. This information also allows the geologist to understand complexities and heterogeneities that exist at all scales. Core-based measurements incorporate a wide range of tools that cover all scales from visual core inspection, petrographic thin section analysis, nanometer scanning electron microscopy, and high-resolution X-ray fluorescence (XRF) chemofacies characterizations. In addition to core descriptions, rock and fluid attributes (i.e., mineralogy, oil and water saturation, rock mechanical properties, porosity, and permeability) are measured or imaged at specific core locations that are often widely spaced across the core. Integrating all of these measurements, observations, learnings, and characterizations into a unified framework that can be applied to all cores across a formation of interest remains a challenge. Calibrating wireline logs from core-based measurements has been a particular challenge due to the low spatial resolution of key rock- and fluid-attribute measurements. This challenge prevents geologists and petrophysicists from applying the findings from one core to all cores and wireline logs within the basin.
The objective of CorePy is to integrate all core-based measurements into a unified framework that captures key core-based chemofacies and lithofacies characterizations for the formation of interest, and ties rock- and fluid-attribute measurements to those chemofacies. This integrative approach is based on high-resolution (2 inch) XRF measurements assembled into formation-specific training data sets that are evaluated using a combination of supervised visual lithofacies clusters and unsupervised chemofacies clusters. A deep neural network model is trained on this formation-specific training data set to classify the statistical probability and spatial distribution of chemofacies across all cores in the region of interest. Measured rock and fluid attributes are integrated into the model to further characterize each chemofacies. The chemofacies stacking pattern within each core is then used to model mineralogy, grain density, porosity, and water saturation curves that can be tied directly to wireline logs for upscaling.
Currently the MSRL hosts trained chemofacies models for the Eagle Ford Group, Wolfcamp Formations across Midland and Delaware Basins, the Bone Spring Formation across Delaware Basin, and the Vaca Muerta Formation in Argentina. New research is applying these models to the Barnett Shale, the Marcellus Formation, the Bakken Formation, and the Austin Chalk.
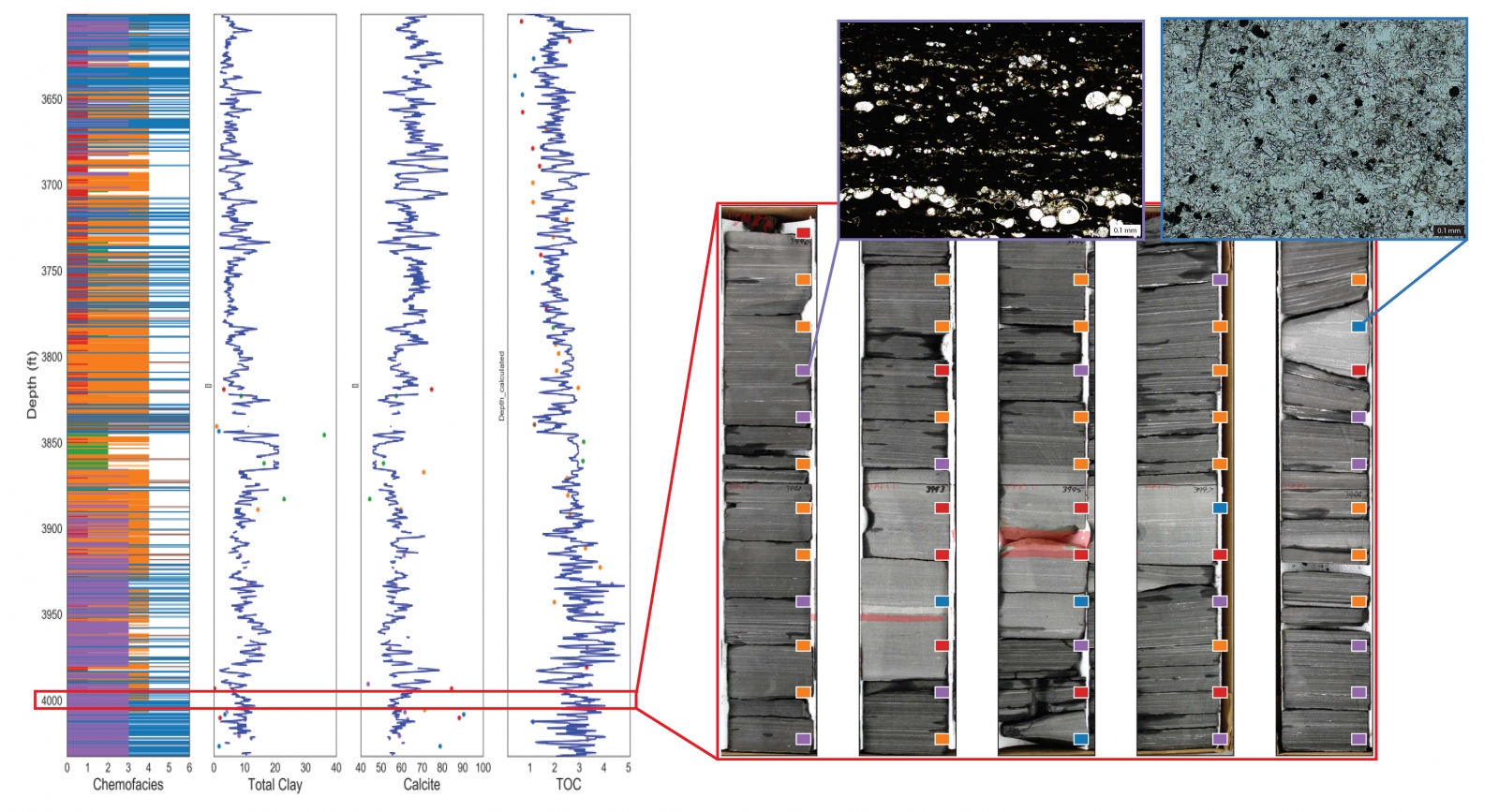
Figure 1. By integrating high-resolution XRF core measurements with mineralogy and organic carbon measurements from core plugs using neural network modeling, MSRL researchers can upscale core-based measurements to wireline logs. CorePy adds visualization so researchers can better understand chemostratigraphic stacking patterns and tie them to thin-section and SEM images.
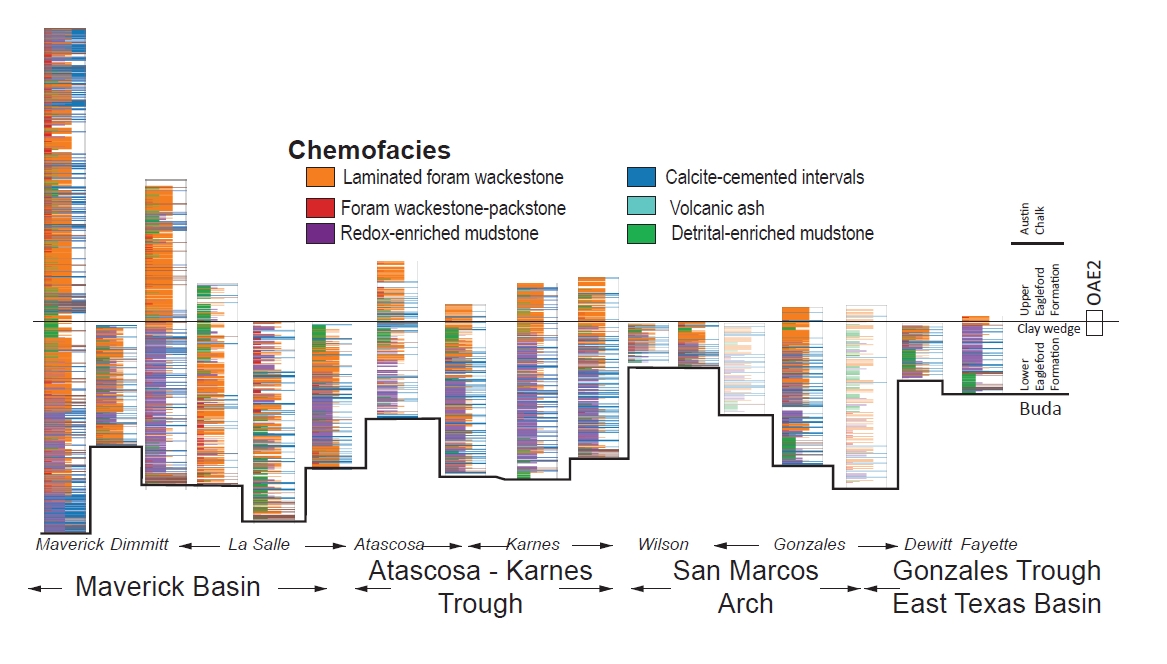
Figure 2. Neural network chemofacies predictions from CorePy for 17 Eagle Ford Group cores across the Maverick Basin, Karnes Trough, and the San Marcos Arch. Upscaling of core-based chemofacies is used to address spatial distribution of key rock and fluid attributes including mineralogy, total organic carbon, porosity, and water saturation.
Research Program: Mudrock Systems Research Laboratory (MSRL)
Project dates: March 2019–January 2022
Project PI: Toti Larson
Other key personnel: Evan Sivil, Lucy Tingwei Ko, and Priyanka Periwal
Principal information contact:
Toti Larson
Email: toti.larson@beg.utexas.edu
Telephone: (512) 838-3230
Funding source: MSRL consortia members
Other key partners/collaborators: Jonathan Knapp (Bruker Nano Analytics), Robert Reed (MSRL)
Geographic areas of study: Eagle Ford Shale, Bone Spring Formation, Wolfcamp Formation, Vaca Muerta Formation
General disciplines: Machine learning, sedimentary depositional systems, facies interpretation and core characterizations, informing wireline logs and basin models, data analytics
Keywords: Eagle Ford Group, machine learning, Wolfcamp Formation, Bone Spring Formation, mudrock systems