About Yangkang Chen
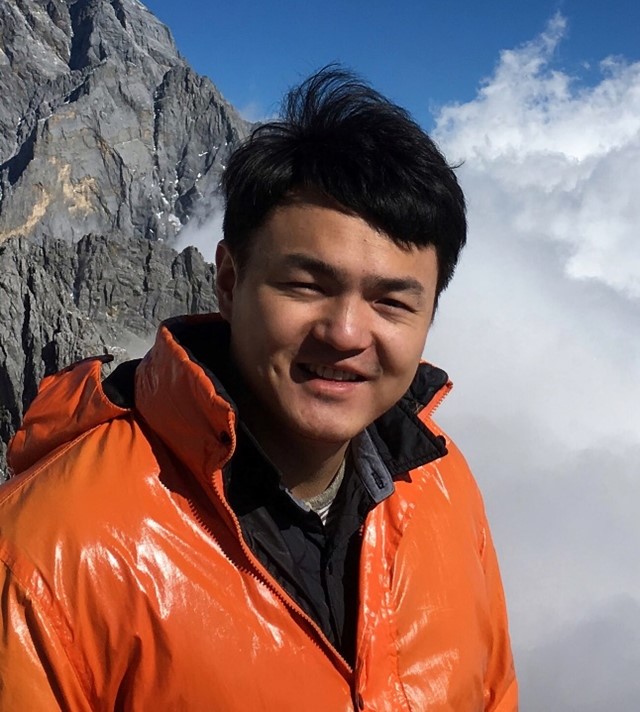
Yangkang Chen is a seismologist at TexNet at the Bureau of Economic Geology. He received a B.S. degree in exploration geophysics from China University of Petroleum in Beijing, China, in 2012 and a Ph.D. degree in geophysics from The University of Texas at Austin in 2015. From 2016 to 2018, he was a distinguished postdoctoral research associate with the Oak Ridge National Laboratory in Oak Ridge, Tennessee. His research interests include seismic signal analysis, seismic modeling and inversion, deep learning, and high-performance computing.
What are your current research activities?
My current research focuses on applying artificial intelligence (AI) techniques to a variety of geoscience problems. One particularly challenging problem is to detect earthquakes that are likely induced by industrial injection activities from continuous waveforms of hundreds of broadband stations, which requires a precise picking of key seismic phases (e.g., P and S waves) and a sophisticated workflow for the association and location of the detected phases. AI really plays an important role in this complicated workflow.
What excites you the most about your current research?
Everyone knows AI, and everyone knows AI can be helpful in their own field. But not all know exactly how fascinating AI can be in geoscience. In my everyday research, I am impressed again and again by the amazing and unbelievable job AI does. Only when you dig into the fundamentals of AI, and apply it just right, can you find out what unprecedented things AI can do that humans cannot. That is what I am most excited about—I am witnessing the revolutionary change AI is making in my field.
What is the desired outcome of your research?
My desired outcome is the methodological breakthrough in various seismological, or more broadly geoscientific, problems, in the forms of research papers and open-source software.
What do you need in order to make your research efforts more successful?
To achieve breakthroughs in our field by leveraging the power of AI, I need to dig into the fundamentals of all problems we study. I need to deeply understand the principles of all state-of-the-art approaches, most importantly their limitations, so that I can design new approaches with the help of AI to tackle the true issues existing within the current methods. Throughout the development process, I need to collaborate continuously with domain experts.
What are your latest papers or publications, and what is most exciting about them?
My latest paper is about an open-source package called pyekfmm, which was developed for traveltime calculation, a fundamental problem in all areas of seismology.
The pyekfmm package offers a seamless compilation of the backbone C programs in the Python environment through a state-of-the-art pip installation. As a result, users can use the pyekfmm package for different scientific purposes with the convenience enabled by Python interfaces and with the efficiency offered by C programs.
Who will benefit from your latest paper or publication?
All seismologists that utilize Python may benefit from this package, especially for those beginner seismologists seeking educational resources. Our package is fully open-source, easy to install, and published with numerous reproducible experiments; thus it facilitates education and research development.
What was your most exciting paper or publication and why?
My most exciting paper is one that is still under review and hopefully coming out in a couple of months. Its title is “EQCCT: A production-ready EarthQuake detection and phase-picking method using the Compact Convolutional Transformer,” which I coauthored with a former postdoc in my group and a team of scientists within the Bureau. The paper includes years of effort from the whole team on the topic of earthquake detection using AI.
In this paper, we proposed an advanced earthquake detection and phase-picking method based on the new deep learning architecture of compact convolutional transformers (EQCCT), which outperforms state-of-the-art AI pickers in various statistical metrics. More importantly, we have applied EQCCT in near real-time earthquake monitoring at TexNet due to its high picking accuracy, which is revolutionary progress in the AI-picker field.
Who are the types of research partners you are seeking, and what skills or expertise could be of benefit to your research?
I would benefit from research partners that share the same research vision as mine. It is not limited to geoscientists that are interested in scientific computation but also mathematicians and physicists that are interested in geoscientific problems.
What are the desired relationships, expertise, or skills that could be brought in to benefit your research?
Our fundamental research goal is to advance seismological studies by promoting open-source scientific research. The users of our open-source software will help us improve the software and update the key methodologies behind the interface of the software. The users of our open-source products are mostly forefront scientific researchers (from companies, universities, national labs, etc.) from around the world, who truly can help us in strengthening our research.
What have been recent successes associated with your research?
Several noteworthy successes are listed below:
- We developed cutting-edge earthquake detection tools for monitoring induced seismicity in the state of Texas.
- We developed a suite of data processing packages for tackling the strong noise in distributed acoustic sensing seismic data sets.
- We developed open-source packages for bridging the gap between exploration and earthquake seismology by making the tools from both communities easily accessible.
- We developed an AI-based method for forecasting the occurrence of future destructive earthquakes, with an overall success rate above 70 percent.
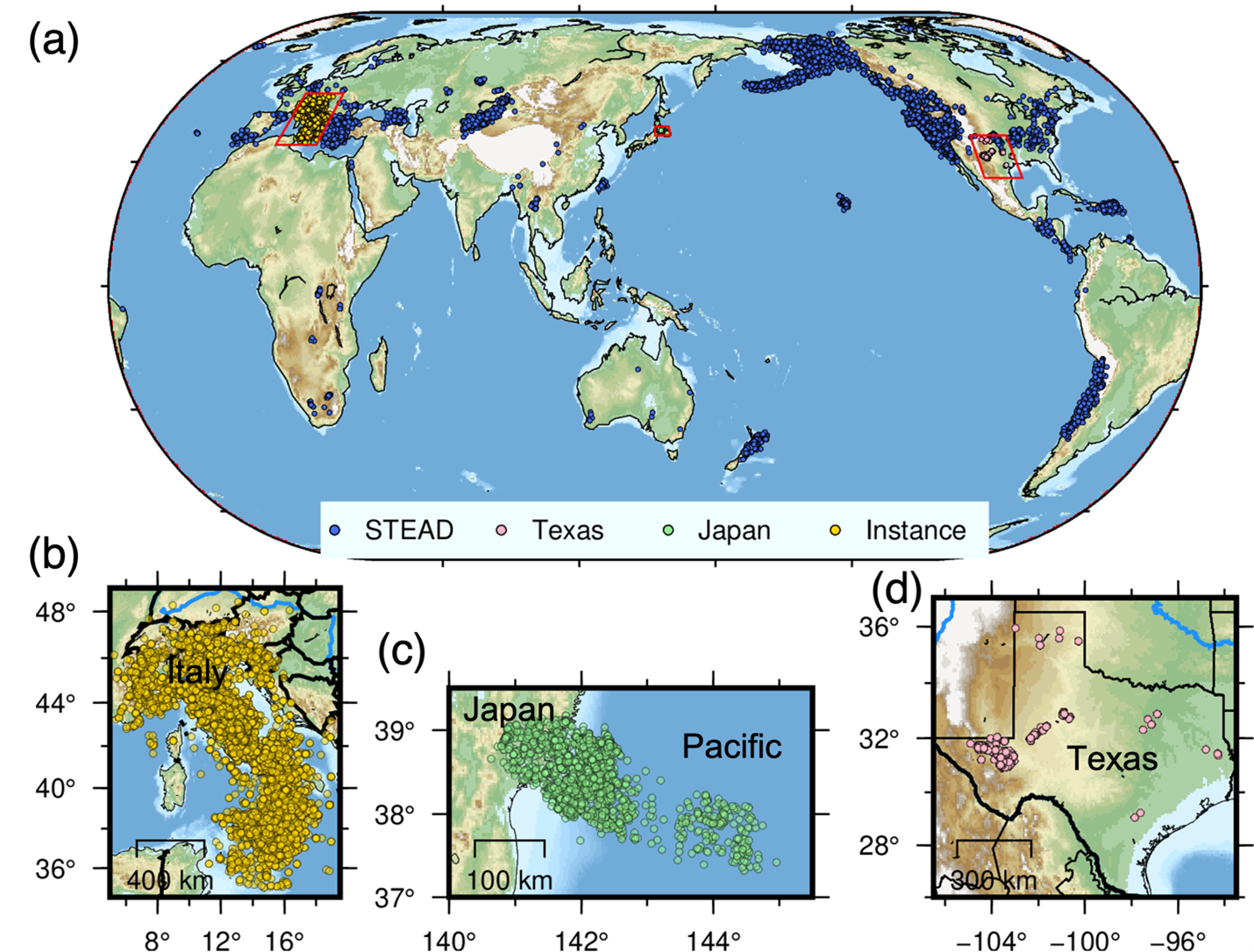
Figure 1. (a) Global, (b) Italy, (c) Japan, and (d) Texas data sets that confirm the highly generalized performance of our AI-based earthquake detection method (EQCCT).
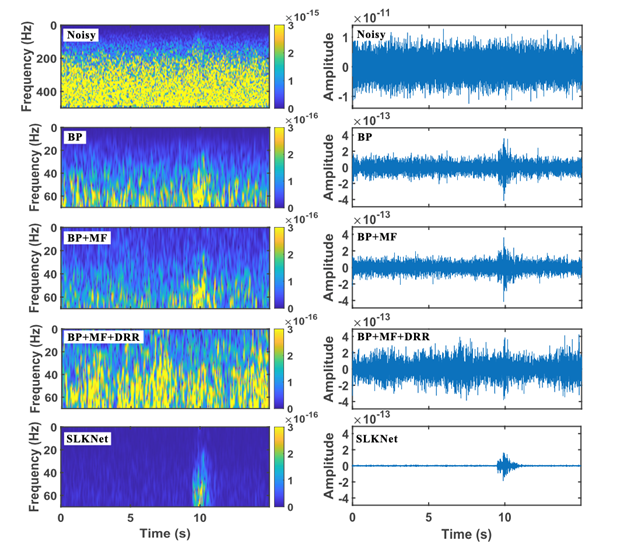
Figure 2. Advanced AI-based processing method for dramatically improving the distributed acoustic sensing seismic data, from the noisy data (top) to the denoised data (bottom). The second to fourth rows correspond to three traditional methods. Left column shows the time-frequency spectra of the right column.
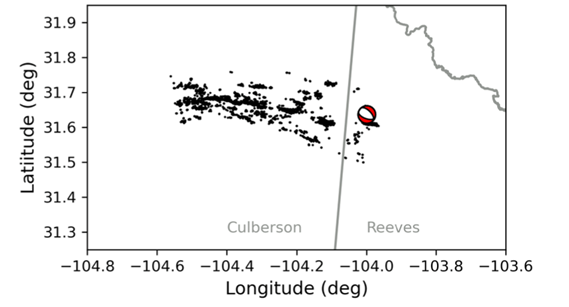
Figure 3. Induced seismicity and fault structure delineated through the AI-picker EQCCT using one-month waveform data.